Betting
Mathematical Models in Betting: How to Apply Analysis for Prediction
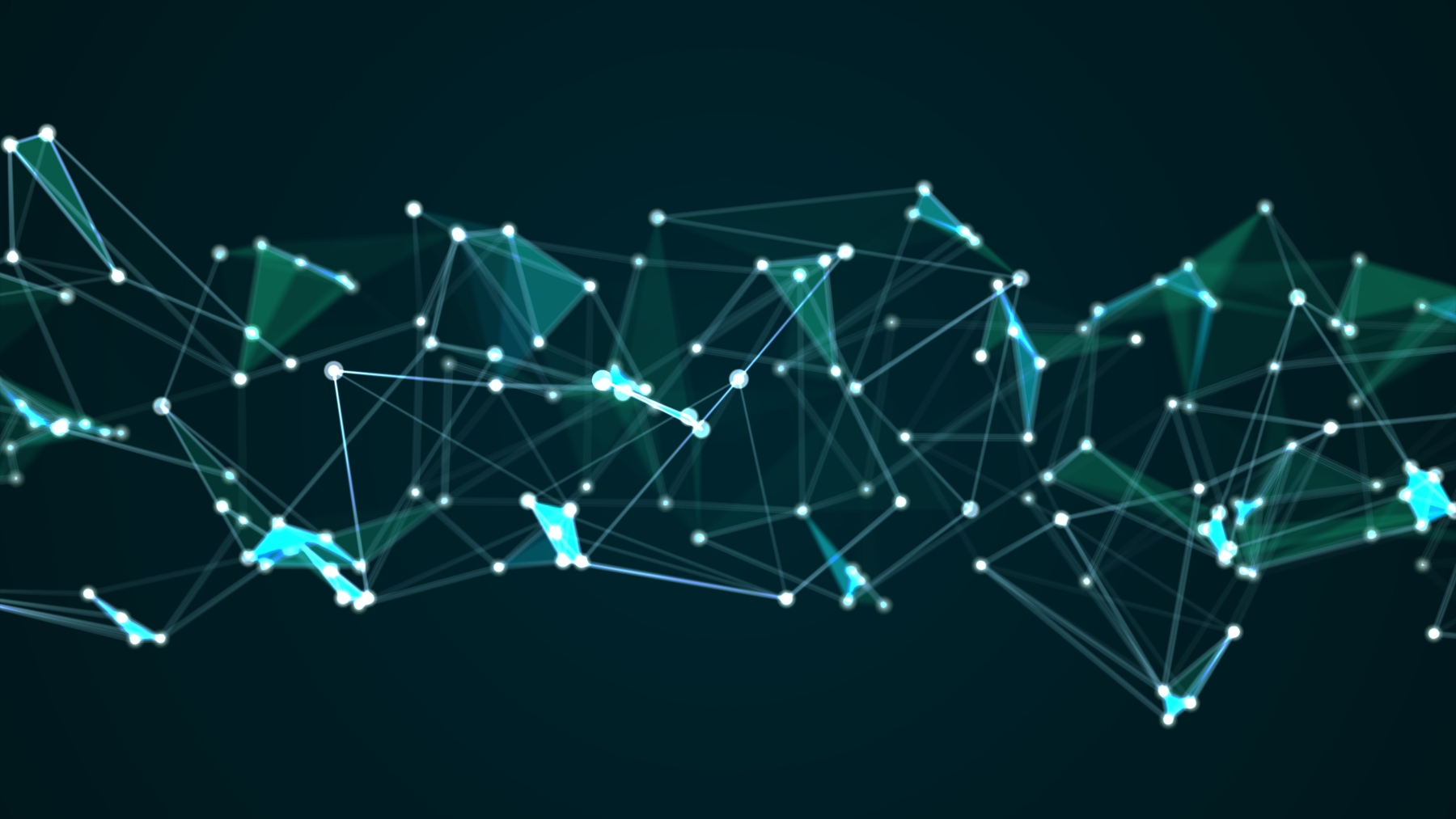
Betting has evolved over the years from a simple leisure activity to a realm where science and mathematics intersect to enhance decision-making. Mathematical models and statistical analysis have become essential tools for bettors seeking an edge in predicting the outcomes of various sporting events, including the dynamic realm of live betting. In this article, we delve into the world of mathematical concepts in punting, exploring how analysis can be applied to forecast outcomes, make informed decisions, and potentially increase the chances of success.
Understanding Mathematical Models in Betting
Mathematical models are structured representations of real-world situations that use analytical equations and variables to describe and predict the behavior of a phenomenon. In the context of punting, these concepts attempt to capture the underlying factors that influence the outcomes of events, such as sports matches, horse races, or casino games. These math patterns rely on historical data, statistical patterns, and various parameters to make predictions.
Types of Mathematical Models
1. Probability
Probability models are fundamental to the betting analysis. They involve calculating the likelihood of different outcomes occurring based on historical data and relevant variables. These concepts help bettors quantify the risks and potential rewards of their wagers. One common example is the Poisson distribution, often used to predict the number of goals scored in a football match.
2. Regression
Regression concepts explore the relationships between various variables and outcomes. Linear regression, for instance, assesses how changes in one variable correspond to changes in another. Bettors can use regression analysis to identify correlations and trends that may offer insights into predicting outcomes, such as a player’s performance based on factors like weather conditions or historical data.
3. Machine Learning
Machine learning techniques are widely used in punting analysis due to their ability to process huge amounts of data and identify complex patterns. Algorithms like decision trees, neural networks, and support vector machines can analyze multiple variables simultaneously, enabling bettors to generate more accurate predictions. These models can be particularly useful for identifying hidden insights in complex betting scenarios.
Applying Mathematical Models for Prediction
1. Data Collection and Preparation
The first step in applying analytical patterns to betting is data collection. Historical data on relevant variables, such as team performance, player statistics, weather conditions, and venue specifics, are essential. Once collected, the data needs to be cleaned, organized, and standardized to ensure accuracy and consistency.
2. Selection
Choosing the appropriate concept depends on the specific sporting event and the available data. For example, if you are analyzing race results, a regression model is suitable for predicting the winning horse based on various factors such as jockey experience, track condition, and previous race results.
3. Parameter Estimation
Model parameters are the coefficients or values that the concept uses to make predictions. Estimating these parameters requires optimization techniques that involve finding the values that minimize the difference between the predicted outcomes and the actual results from historical data.
4. Validation and Testing
Validating and testing the pattern’s accuracy is crucial. Historical data can be divided into training and testing sets. The concept is trained using the training set and then tested on the testing set to evaluate its predictive performance. Cross-validation techniques, such as k-fold cross-validation, help ensure the model’s reliability.
5. Refinement and Iteration
Models are not static; they can be refined and improved based on feedback from testing and validation. Adjusting concept parameters, incorporating additional variables, or fine-tuning algorithms can lead to better predictive accuracy.
Challenges and Considerations
1. Overfitting and Underfitting
Overestimation occurs when a model performs well on training data but poorly on new, unknown data. On the other hand, underestimation results when the concept fails to capture the patterns underlying the data. To avoid these problems, it is necessary to find the right balance between complexity and simplicity.
2. Data Quality and Availability
The accuracy of math patterns is highly dependent on the quality and quantity of data available. Incomplete or biased data can lead to inaccurate forecasts. In addition, for some events on which bets are placed, complete historical information may not be available, making accurate modeling difficult.
3. Changing Dynamics
Event betting is subject to various external factors that can change the dynamics of an event. These factors, such as player injuries or unforeseen weather conditions, may not be adequately accounted for in the models.
Conclusion
Math patterns and statistical analysis have revolutionized the punting world, allowing bettors to make more informed predictions and strategic decisions. The application of analytical concepts can extract valuable insights from historical data and relevant variables. However, it’s essential to recognize the limitations of these models and consider the ever-changing nature of betting events. By embracing mathematical modeling and applying it judiciously, bettors can harness the power of data-driven analysis to increase their chances of success.
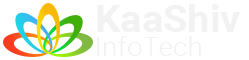
Betting
The Surge of Mobile Betting Apps in India
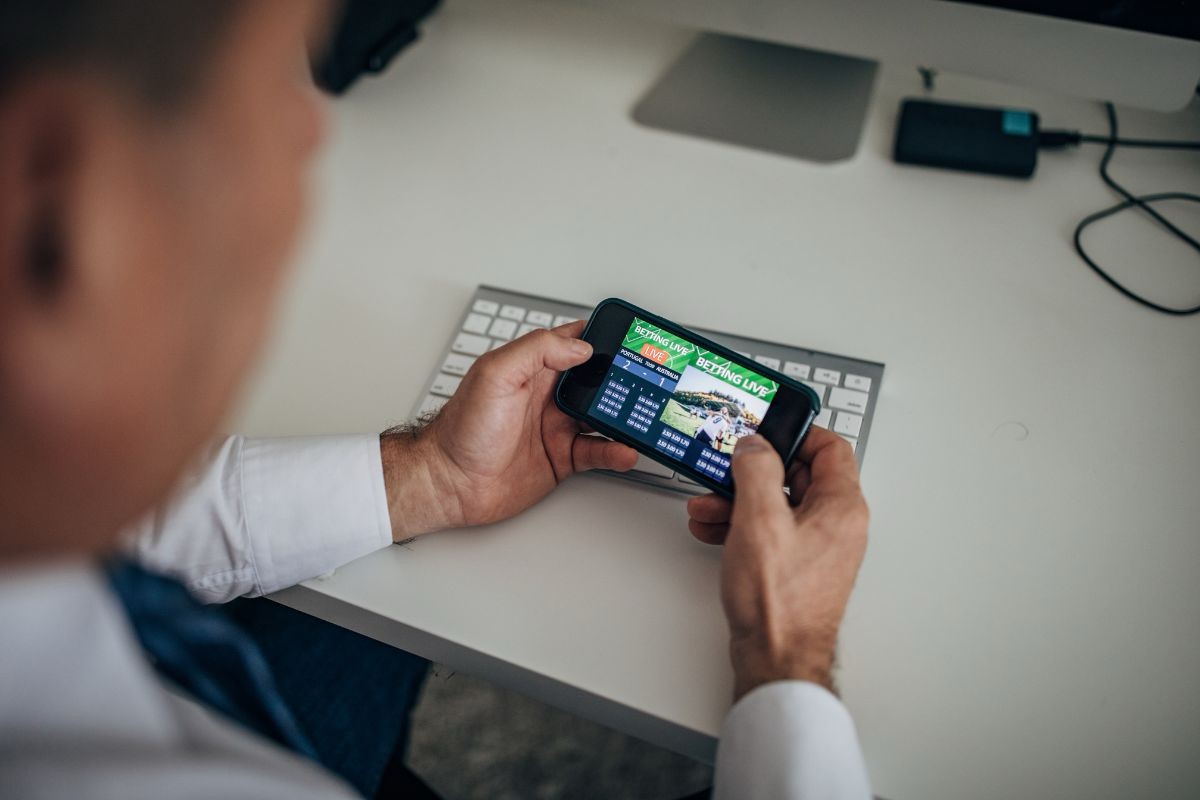
In recent years, India has witnessed a significant surge in the popularity of betting through mobile bookmaker apps. This phenomenon has transformed the landscape of betting in the country, introducing an era where convenience and accessibility are at the forefront. The advent of mobile technology has played a pivotal role in demystifying betting, making it more approachable and enjoyable for a broader audience.
Enhanced User Experience
One of the most apparent benefits of mobile betting apps is the enhanced user experience they offer. With intuitive registration and seamless navigation, these apps cater to both seasoned bettors and newcomers alike. Users can place bets, track live scores, and manage their accounts with utmost ease, without the need to be tethered to a desktop computer. This level of convenience has not only attracted a new wave of users but has also enriched the betting experience for existing enthusiasts.
Accessibility and Convenience
The rise of mobile betting apps in India has brought about unparalleled accessibility and convenience. Whether at home, in a café, or on the move, users can engage with their favorite sports and events at the touch of a button. This omnipresence of betting opportunities has encouraged more people to explore betting, significantly expanding the community of bettors across the country. The ability to bet anytime and anywhere has truly revolutionized the way people interact with sports betting.
A Boost in Engagement
Mobile bookmaker apps have significantly boosted engagement within the betting community. Through features like live betting, instant notifications, and social sharing, these apps offer a dynamic and interactive experience that keeps users engaged. The excitement of placing live bets on cricket matches, football games, and other sports has heightened interest and participation in betting activities. Additionally, the community aspect fostered by app platforms has created a sense of camaraderie among users, further fueling their enthusiasm.
Safe and Secure Betting Environment
Safety and security are paramount concerns for bettors, and mobile betting apps in India have addressed these concerns effectively. With advanced encryption technologies and secure payment gateways, these apps provide a safe environment for users to place bets confidently. This assurance of security has played a critical role in attracting users who were previously hesitant about online betting. Knowing that their personal and financial information is protected, users can focus on enjoying the betting experience.
The Impact on the Betting Market
The proliferation of mobile betting apps has had a profound impact on the betting market in India. It has broadened the market’s reach, bringing betting to a more diverse audience. The competitive landscape has encouraged innovation, leading to improved services and features that benefit users. This healthy competition has led to better odds, more betting options, and enhanced promotional offers, all of which contribute to a vibrant and thriving betting ecosystem.
Top Sports for Mobile Betting in India
In India, cricket is not just a sport; it’s a religion. This immense passion for cricket naturally extends into the realm of betting, making it the sport in the greatest demand through mobile applications of bookmakers. The Indian Premier League (IPL), with its thrilling matches and galaxy of international stars, stands out as the pinnacle event for bettors. Additionally, international tournaments like the ICC Cricket World Cup and bilateral series also see substantial betting activity. The ability to bet on various outcomes, including match winners, top scorers, and even specific ball-by-ball actions, makes cricket betting incredibly engaging for users.
Football’s Growing Foothold
While cricket leads the pack, football betting is quickly gaining traction among Indian bettors. The Indian Super League (ISL) has played a significant role in this surge by elevating the standard of football in the country. Beyond domestic leagues, international competitions such as the FIFA World Cup, UEFA Champions League, and the English Premier League attract a considerable number of bets. The dynamic nature of football, with its global fan base and unpredictable outcomes, makes it a favorite for those looking to add excitement to their viewing experience.
The Rising Star of Kabaddi
Kabaddi has made remarkable strides in terms of popularity, thanks in large part to the Pro Kabaddi League (PKL). This homegrown sport has found a new lease of life, capturing the imagination of the masses and, by extension, the betting community. Mobile betting apps have recognized this trend, offering users the opportunity to bet on their favorite teams and players. The sport’s fast-paced action and the patriotic fervor it evokes make it a unique offering in the betting market.
Tennis – A Year-Round Favorite
Tennis betting holds a special place in the hearts of Indian bettors, primarily due to the sport’s year-round calendar. Grand Slam tournaments, namely the Australian Open, French Open, Wimbledon, and the US Open, are particularly popular for betting. The individual nature of the sport allows for a wide array of betting options, from match winners and set scores to more nuanced bets like first serve percentages and break points saved. The global stature of these tournaments and the presence of tennis icons enhance the appeal of tennis betting.
Horse Racing – The Classic Choice
Horse racing, one of the oldest forms of sports betting, continues to maintain its charm and popularity among Indian bettors. The sport offers a blend of tradition, glamour, and excitement, making it a perennial favorite for wagering through mobile apps. Prestigious events like the Indian Derby and the Indian 1000 and 2000 Guineas attract significant betting volumes. The unpredictability and the rich history of horse racing contribute to its enduring allure.
The Rise of Mobile Casino Gaming in India
In the bustling digital landscape of India, mobile applications of bookmakers have expanded their horizons to include a diverse range of casino games. This integration has captured the interest of enthusiasts across the nation, creating a vibrant and dynamic online gaming ecosystem. With the convenience of mobile technology, users can now indulge in their favorite casino games anytime and anywhere, leading to a surge in popularity of certain games.
The Reign of Slot Machines
Slot machines stand as the uncontested leaders in mobile casino gaming within India. Their simplicity, coupled with the allure of instant wins, has made them a go-to option for many players. Slots come in various themes and configurations, from classic three-reel games to complex multi-line video slots featuring elaborate themes and bonus rounds. The appeal lies in their straightforward gameplay, where bets can range from a few cents to several hundred rupees per spin, accommodating all levels of risk appetite.
The Strategic Appeal of Blackjack
Following closely is blackjack, a game that combines luck with strategic decision-making. Indian players appreciate the challenge it presents, requiring not just chance but thoughtful consideration of every hand. Bets in blackjack can vary, including:
- Standard bets on the player’s hand beating the dealer’s.
- Side bets like ‘Perfect Pairs’ and ’21+3′, which offer higher payouts for specific combinations.
- Insurance bets, where players can guard against the dealer hitting blackjack.
This variety keeps the game engaging and allows for different betting strategies, making blackjack a staple in the mobile casino gaming scene.
Roulette’s Diverse Betting Options
Roulette has also captured the fascination of Indian bettors, thanks to its wide array of betting options and the suspense of the spinning wheel. Players can place bets on:
- A single number for high payouts.
- Various combinations of numbers.
- Colors (red or black).
- Odd or even numbers.
- Different sections of the wheel.
These options provide flexibility and control over the level of risk, appealing to both cautious players and high rollers.
The Thrill of Live Dealer Games
Live dealer games have introduced a new dimension to mobile casino gaming, offering an immersive experience that replicates the atmosphere of a land-based casino. Popular live dealer games include variations of poker, baccarat, and roulette, allowing players to interact with real dealers and other players in real-time. The types of bets mirror those of their physical counterparts, enriching the gaming experience with a social element and the excitement of live action.
Poker’s Enduring Popularity
Poker remains a favored choice among Indian players, with Texas Hold’em leading the pack. The game demands skill, psychological insight, and strategy, drawing players who seek more than just luck-based gaming. The betting dynamics in poker are intricate, involving:
- Ante bets to start the round.
- Call, raise, and fold decisions based on hand strength and opponents’ actions.
- Pot-limit and no-limit betting structures, offering a range of strategies and risk levels.
Poker’s complexity and the influence of player skill make it a staple in the mobile gaming community.
Leading Mobile Bookmaker Applications in India
In the dynamic world of online betting, mobile applications have become the gateway to placing bets on a myriad of sports and casino games. In India, the enthusiasm for such platforms is on a significant rise, with several key players dominating the market. Among them, Melbet, Dafabet, 1Win, and 12bet have established themselves as favorites, each offering unique advantages to their users.
Melbet – A Versatile Betting Platform
Melbet distinguishes itself with an extensive range of betting options, covering a vast array of sports from cricket, football, to niche sports. The application boasts a user-friendly interface, making navigation and placing bets straightforward for both beginners and seasoned bettors. One of its standout features is the live betting option, allowing users to place bets on ongoing events, complemented by live-streaming of select matches, enhancing the overall betting experience. Additionally, Melbet offers competitive odds, ensuring that users get great value from their bets.
Parimatch – Innovation and User Engagement
Parimatch has rapidly gained popularity for its innovative approach to online betting. The application features a sleek design and an intuitive user interface, facilitating easy access to a wide range of sports and casino betting options. It stands out for its promotional offers and bonuses, specifically designed to enhance user engagement and loyalty. Furthermore, Parimatch places a strong emphasis on customer service, providing comprehensive support to ensure user satisfaction. The addition of virtual sports betting is another feature that sets Parimatch apart, offering users a unique betting avenue.
1Win – Competitive Odds and Broad Market Coverage
1Win makes its mark with some of the most competitive odds in the industry, particularly appealing to those looking to maximize their winnings. It covers a broad spectrum of sports, including popular and lesser-known events, ensuring there’s something for every type of bettor. The app is commendable for its straightforward deposit and withdrawal processes, offering a range of payment methods suitable for Indian users. 1Win also prides itself on its efficient customer support system, ready to assist users with any queries or issues.
12bet – Focus on Sports and Live Betting
12bet is primarily known for its strong focus on sports betting, offering extensive markets for a variety of sports including cricket, football and tennis. The app is great for live betting, providing real-time updates and odds that make betting fun. Its user-friendly interface is designed for both beginners and experienced players, providing easy navigation. 12bet also offers competitive bonuses and promotions, as well as easy 12bet registration, designed to reward regular users and attract new ones.
Betting
Why 4RaBet is the Best Betting Website in India
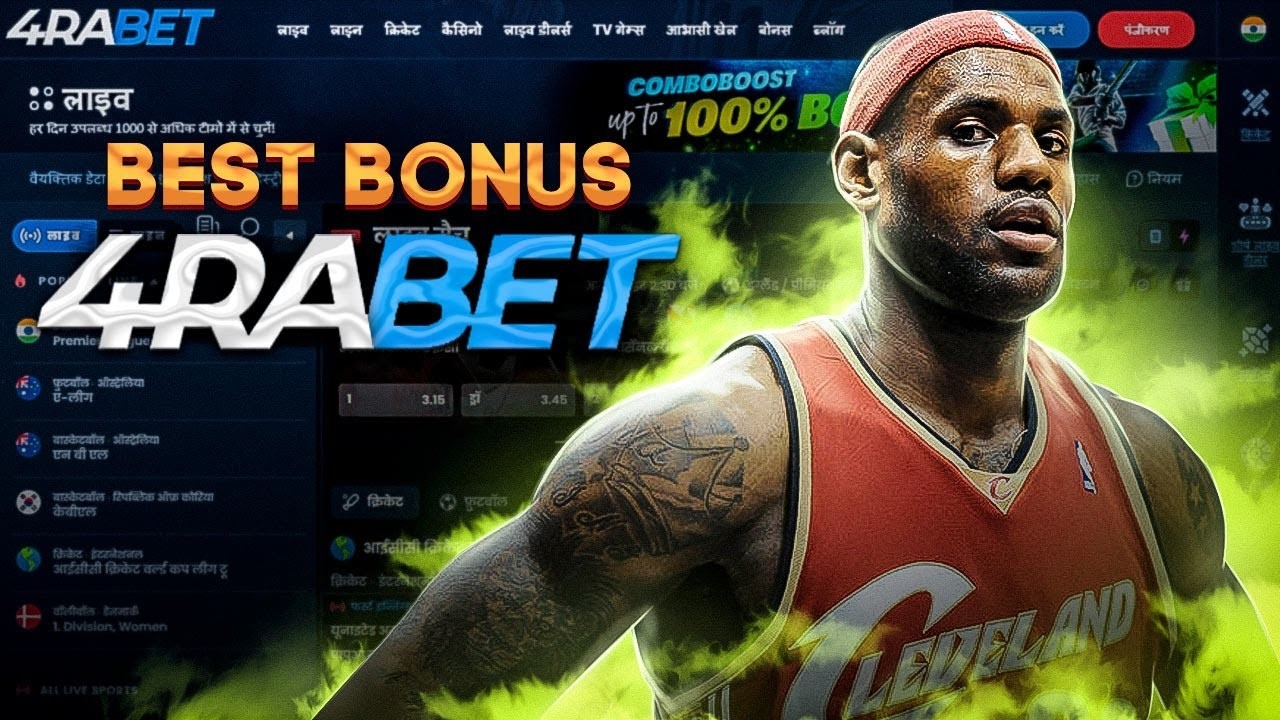
Introduction: In the bustling landscape of online betting platforms in India, 4rabet has emerged as a shining beacon of excellence, captivating bettors with its stellar features, unparalleled services, and commitment to providing a top-tier betting experience. As a seasoned expert in the field of betting, I’ve meticulously analyzed the key factors that set 4rabet-india.in apart from its competitors, and in this article, I’ll unveil why it stands out as the best betting site in India, backed by concrete evidence and undeniable proof.
Aspect | Description |
Wide Range of Sports | 4rabet offers a diverse selection of sports including cricket, football, tennis, basketball, and more. |
Extensive Betting Markets | Bet on a wide range of markets including pre-match and live betting options for maximum flexibility. |
Competitive Odds | Enjoy competitive odds across various sports and markets, ensuring maximum value for your bets. |
User-Friendly Interface | 4rabet provides a sleek and intuitive interface for seamless navigation and efficient betting. |
Robust Security Measures | Rest assured knowing your personal and financial information is protected by robust security measures. |
Responsible Gambling Practices | 4rabet promotes responsible gambling with tools and resources to help bettors gamble responsibly. |
Exceptional Customer Support | Access 24/7 customer support via live chat, email, or phone for prompt assistance and resolution. |
Wide Range of Sports and Markets
One of the hallmarks of a premier betting site is its extensive coverage of sports and betting markets, and 4rabet excels in this regard. With a diverse selection of sports ranging from cricket and football to tennis, basketball, and beyond, 4rabet caters to the preferences of every bettor, ensuring there’s something for everyone. Moreover, its comprehensive range of betting markets, including pre-match and live betting options, allows bettors to explore a plethora of betting opportunities and maximize their potential returns.
Competitive Odds and Favorable Margins
In the fiercely competitive world of online betting, odds play a pivotal role in determining the value and profitability of bets. 4rabet distinguishes itself by offering competitive odds across a wide range of sports and markets, ensuring bettors receive the best possible returns on their investments. Furthermore, its favorable margins and low overrounds give bettors a significant advantage compared to other betting sites, making 4rabet the go-to destination for savvy bettors seeking maximum value.
User-Friendly Interface and Seamless Navigation
Navigating an online betting platform should be a seamless and intuitive experience, and 4rabet excels in providing a user-friendly interface that caters to the needs of both novice and experienced bettors alike. With its sleek design, responsive layout, and easy-to-navigate menus, 4rabet ensures bettors can place bets quickly and efficiently without any hassle. Moreover, its mobile-friendly platform allows bettors to enjoy their favorite sports and betting markets on the go, providing unparalleled convenience and accessibility.
Robust Security Measures and Responsible Gambling Practices
When it comes to online betting, security and safety are paramount, and 4rabet prioritizes the protection of its users’ personal and financial information through robust security measures and encryption protocols. Additionally, 4rabet is committed to promoting responsible gambling practices and provides a range of tools and resources to help bettors manage their betting activity responsibly. From setting deposit limits to self-exclusion options, 4rabet empowers bettors to gamble responsibly and enjoy a safe betting environment.
Exceptional Customer Support and Reliability
Last but not least, 4rabet prides itself on delivering exceptional customer support and reliability, ensuring bettors receive prompt assistance and resolution to any queries or issues they may encounter. With its dedicated customer support team available 24/7 via live chat, email, and phone, bettors can rest assured knowing that help is always just a click or call away. Moreover, 4rabet’s reliability and reputation for transparency and fairness have earned it the trust and loyalty of bettors across India.
Conclusion: In conclusion, 4rabet stands head and shoulders above the competition as the premier betting site in India, offering an unparalleled betting experience characterized by extensive sports coverage, competitive odds, user-friendly interface, robust security measures, responsible gambling practices, and exceptional customer support. With its commitment to excellence and dedication to customer satisfaction, 4rabet continues to raise the bar in the online betting industry and remains the top choice for discerning bettors seeking the ultimate betting experience in India.
-
Internet4 years ago
How to Download a Copy of Your Aadhaar Card
-
Sports2 years ago
10Cric India Bookmaker Review for Betting on Sports Online in 2022
-
Apps4 years ago
How to Book an Ola or Uber Using Google Maps
-
Android Games4 years ago
LDPlayer vs Nox Player: Powerful Android Emulator for Gaming
-
Apps4 years ago
How to Install WhatsApp Beta for Windows Mobile or Windows Phone
-
How to4 years ago
Jio Fiber Landline Service: How to Activate Jio Home Phone aka JioFixedVoice for Free Calling
-
How to4 years ago
WhatsApp Web: Everything You Need to Know
-
How to4 years ago
How to Increase Followers on Instagram for Real